Abstract
The ability to ‘sense’ the social environment and thereby to understand the thoughts and actions of others allows humans to fit into their social worlds, communicate and cooperate, and learn from others’ experiences. Here we argue that, through the lens of computational social science, this ability can be used to advance research into human sociality. When strategically selected to represent a specific population of interest, human social sensors can help to describe and predict societal trends. In addition, their reports of how they experience their social worlds can help to build models of social dynamics that are constrained by the empirical reality of human social systems.
Access options
Subscribe to Journal
Get full journal access for 1 year
199,00 €
only 3,90 € per issue
Tax calculation will be finalised during checkout.
Rent or Buy article
Get time limited or full article access on ReadCube.
from$8.99
All prices are NET prices.
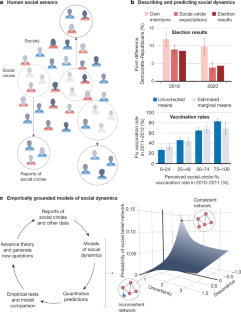
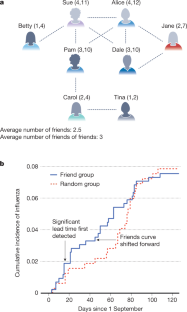
References
- 1.
Tomasello, M. Becoming Human: A Theory of Ontogeny (Belknap, 2019).
- 2.
Galesic, M. et al. Asking about social circles improves election predictions. Nat. Hum. Behav. 2, 187–193 (2018). Shows that human social sensing outperformed traditional polling questions in forecasting for the 2016 US and 2017 French elections.
- 3.
Olsson, H., Bruine de Bruin, W., Galesic, M. & Prelec, D. Election polling is not dead: a Bayesian bootstrap method yields accurate forecasts. Preprint at https://doi.org/10.31219/osf.io/nqcgs (2021). Developed an information integration method that provided accurate forecasts of the 2018 and 2020 US elections by combining own intentions with human social sensor reports.
- 4.
Bruine de Bruin, W. et al. Asking about social circles improves election predictions even with many political parties. Preprint at https://doi.org/10.31219/osf.io/8g5ce (2021). Shows that human social sensing outperformed traditional polling questions in forecasting for the 2017 Dutch and 2018 Swedish elections.
- 5.
Bruine de Bruin, W., Parker, A. M., Galesic, M. & Vardavas, R. Reports of social circles’ and own vaccination behavior: a national longitudinal survey. Health Psychol. 38, 975–983 (2019). Shows that perceived social circle vaccination coverage helps to predict own future vaccination behaviour.
- 6.
Christakis, N. A. & Fowler, J. H. Social network sensors for early detection of contagious outbreaks. PLoS One 5, e12948 (2010). Shows that asking people about their friends helps to predict outbreaks of contagious diseases.
- 7.
Graefe, A. Accuracy of vote expectation surveys in forecasting elections. Public Opin. Q. 78, 204–232 (2014). Shows that the people’s expectations about the election winner help to forecast US elections from 1932 to 2012.
- 8.
Berg, J. E., Nelson, F. D. & Rietz, T. A. Prediction market accuracy in the long run. Int. J. Forecast. 24, 285–300 (2008). Shows that prediction markets outperformed polls in forecasting US elections from 1988 to 2004.
- 9.
Rothschild, D. M. & Wolfers, J. Forecasting elections: Voter intentions versus expectations. SSRN Electron. J. https://doi.org/10.2139/ssrn.1884644 (2011). Shows that accuracy of human social sensing is likely to stem from people’s knowledge about their immediate social environments.
- 10.
Garcia-Herranz, M., Moro, E., Cebrian, M., Christakis, N. A. & Fowler, J. H. Using friends as sensors to detect global-scale contagious outbreaks. PLoS One 9, e92413 (2014). Shows that monitoring the friends of randomly selected Twitter users helps to predict the use of novel hashtags a week earlier than monitoring random users.
- 11.
Galesic, M., Olsson, H., Dalege, J., van der Does, T. & Stein, D. L. Integrating social and cognitive aspects of belief dynamics: towards a unifying framework. J. R. Soc. Interface 18, rsif.2020.0857 (2021). Introduces a unifying framework for modelling both social and cognitive aspects of belief dynamics.
- 12.
van der Does, T., Stein, D. L., Fedoroff, N. & Galesic, M. Moral and social foundations of beliefs about scientific issues: predicting and understanding belief change. Preprint at https://doi.org/10.31219/osf.io/zs7dq (2021). Develops a statistical-physics-inspired model to show that belief change is more likely when educational interventions decrease belief dissonance and at the same time highlight this dissonance.
- 13.
Dalege, J. & van der Does, T. Changing beliefs about scientific issues: the role of moral and social belief networks. Preprint at https://arxiv.org/abs/2102.10751 (2021). Shows that dissonance in one’s reports on moral and social beliefs predicts belief change.
- 14.
Happé, F., Cook, J. L. & Bird, G. The structure of social cognition: in(ter)dependence of sociocognitive processes. Annu. Rev. Psychol. 68, 243–267 (2017).
- 15.
Krueger, J. I. & Funder, D. C. Towards a balanced social psychology: causes, consequences, and cures for the problem-seeking approach to social behavior and cognition. Behav. Brain Sci. 27, 313–327, discussion 328–376 (2004).
- 16.
Ross, L., Greene, D. & House, P. The “false consensus effect”: an egocentric bias in social perception and attribution processes. J. Exp. Soc. Psychol. 13, 279–301 (1977).
- 17.
Chambers, J. R. & Windschitl, P. D. Biases in social comparative judgments: the role of nonmotivated factors in above-average and comparative-optimism effects. Psychol. Bull. 130, 813–838 (2004).
- 18.
Moreno, J. L. Sociometry, Experimental Method and the Science of Society (Beacon House, 1951).
- 19.
Goodman, L. A. Snowball sampling. Ann. Math. Stat. 32, 148–170 (1961).
- 20.
Heckathorn, D. D. Respondent-driven sampling: a new approach to the study of hidden populations. Soc. Probl. 44, 174–199 (1997).
- 21.
Killworth, P. D., Johnsen, E. C., McCarty, C., Shelley, G. A. & Bernard, H. R. A social network approach to estimating seroprevalence in the United States. Soc. Networks 20, 23–50 (1998).
- 22.
Centola, D. How Behavior Spreads: The Science of Complex Contagions (Princeton Univ. Press, 2018).
- 23.
Christakis, N. A. & Fowler, J. H. Connected: The Amazing Power of Social Networks and How They Shape Our Lives (Harper Collins, 2010).
- 24.
Moldoveanu, M. C. & Baum, J. A. C. Epinets: The Epistemic Structure and Dynamics of Social Networks (Stanford Univ. Press, 2014).
- 25.
Keusch, F. & Kreuter, F. in Handbook of Computational Social Science, Volume 1 Theory, Case Studies and Ethics (eds Engel, U., Quan-Haase, A., Xun Lui, S. & Lyberg, L. E.) (Routledge, 2021). Describes different sources of digital trace data and issues related to their use in the computational social sciences, including inferential challenges, measures of reproducibility and replicability, and transparency.
- 26.
Kreuter, F., Haas, G.-C., Keusch, F., Bähr, S. & Trappmann, M. Collecting survey and smartphone sensor data with an app: opportunities and challenges around privacy and informed consent. Soc. Sci. Comput. Rev. 38, 533–549 (2020).
- 27.
Lazer, D. & Radford, J. Data ex machina: introduction to big data. Annu. Rev. Sociol. 43, 19–39 (2017).
- 28.
Varian, H. R. Big data: new tricks for econometrics. J. Econ. Perspect. 28, 3–28 (2014).
- 29.
Feld, S. L. & McGail, A. Egonets as systematically biased windows on society. Netw. Sci. 8, 399–417 (2020). Discusses how the friendship paradox biases the information that people receive from their social environments.
- 30.
Lee, E. et al. Homophily and minority-group size explain perception biases in social networks. Nat. Hum. Behav. 3, 1078–1087 (2019).
- 31.
Lerman, K., Yan, X. & Wu, X.-Z. The “majority illusion” in social networks. PLoS One 11, e0147617 (2016).
- 32.
Chakraborti, A., Toke, I. M., Patriarca, M. & Abergel, F. Econophysics review: II. Agent-based models. Quant. Finance 11, 1013–1041 (2011).
- 33.
Edelmann, A., Wolff, T., Montagne, D. & Bail, C. A. Computational social science and sociology. Annu. Rev. Sociol. 46, 61–81 (2020).
- 34.
Epstein, J. M. Agent_Zero: Toward Neurocognitive Foundations for Generative Social Science (Princeton Univ. Press, 2014).
- 35.
Miller, J. H. & Page, S. E. Complex Adaptive Systems: An Introduction to Computational Models of Social Life (Princeton Univ. Press, 2007).
- 36.
Pentland, A. Social Physics: How Social Networks Can Make Us Smarter (Penguin, 2014). Describes applications of statistical physics and other analogies for modelling complex social systems.
- 37.
Proskurnikov, A. V. & Tempo, R. A tutorial on modeling and analysis of dynamic social networks. Part I. Annu. Rev. Contr. 43, 65–79 (2017).
- 38.
Jung, J., Bramson, A., Crano, W., Page, S. E. & Miller, J. H. Cultural drift, indirect minority influence, network structure and their impacts on cultural change and diversity. Am. Psychol. (in the press).
- 39.
Geanakoplos, J. et al. Getting at systemic risk via an agent-based model of the housing market. Am. Econ. Rev. 102, 53–58 (2012).
- 40.
Hammond, R., Ornstein, J. T., Purcell, R., Haslam, M. D., & Kasman, M. Modeling robustness of COVID-19 containment policies. Preprint at https://doi.org/10.31219/osf.io/h5ua7 (2021).
- 41.
Nowak, A., Szamrej, J. & Latané, B. From private attitude to public opinion: a dynamic theory of social impact. Psychol. Rev. 97, 362–376 (1990).
- 42.
Vallacher, R. R., Read, S. J. & Nowak, A. Computational Social Psychology (Routledge, 2017).
- 43.
Enns, P. K., Lagodny, J. & Schuldt, J. P. Understanding the 2016 US presidential polls: the importance of hidden Trump supporters. Stat. Politics Policy 8, 41–63 (2017).
- 44.
Krumpal, I. Determinants of social desirability bias in sensitive surveys: a literature review. Qual. Quant. 47, 2025–2047 (2013).
- 45.
Wang, D., Szymanski, B. K., Abdelzaher, T., Ji, H. & Kaplan, L. The age of social sensing. Computer 52, 36–45 (2019).
- 46.
Tucker, J. et al. Social media, political polarization, and political disinformation: a review of the scientific literature. SSRN https://doi.org/10.2139/ssrn.3144139 (2018).
- 47.
Smaldino, P. E., Flamson, T. J. & McElreath, R. The evolution of covert signaling. Sci. Rep. 8, 4905 (2018).
- 48.
Alipourfard, N., Nettasinghe, B., Abeliuk, A., Krishnamurthy, V. & Lerman, K. Friendship paradox biases perceptions in directed networks. Nat. Commun. 11, 707 (2020).
- 49.
Sudman, S. & Bradburn, N. M. Asking Questions: A Practical Guide to Questionnaire Design (Jossey-Bass, 1982).
- 50.
Chin, A. & Bruine de Bruin, W. Understanding the formation of consumers’ stock market expectations. J. Consum. Aff. 51, 200–210 (2017).
- 51.
Bruine de Bruin, W., Parker, A. M. & Fischhoff, B. Can adolescents predict significant life events? J. Adolesc. Health 41, 208–210 (2007).
- 52.
Bruine de Bruin, W., Downs, J. S., Murray, P. & Fischhoff, B. Can female adolescents tell whether they will test positive for Chlamydia infection? Med. Decis. Making 30, 189–193 (2010).
- 53.
Hurd, M. D. & McGarry, K. The predictive validity of subjective probabilities of survival. Econ. J. (Lond.) 112, 966–985 (2002).
- 54.
Lewis-Beck, M. S. & Tien, C. Voters as forecasters: a micromodel of election prediction. Int. J. Forecast. 15, 175–184 (1999).
- 55.
Murr, A. E. The wisdom of crowds: what do citizens forecast for the 2015 British General Election? Elect. Stud. 41, 283–288 (2016).
- 56.
Spann, M. & Skiera, B. Internet-based virtual stock markets for business forecasting. Manage. Sci. 49, 1310–1326 (2003).
- 57.
Polgreen, P. M., Nelson, F. D., Neumann, G. R. & Weinstein, R. A. Use of prediction markets to forecast infectious disease activity. Clin. Infect. Dis. 44, 272–279 (2007).
- 58.
Spann, M. & Skiera, B. Sports forecasting: a comparison of the forecast accuracy of prediction markets. J. Forecast. 28, 55–72 (2009).
- 59.
Jussim, L. Social Perception and Social Reality: Why Accuracy Dominates Bias and Self-Fulfilling Prophecy (Oxford Univ. Press, 2012).
- 60.
Brunswik, E. The Conceptual Framework of Psychology (Univ. Chicago Press, 1952).
- 61.
Fiedler, K. Beware of samples! A cognitive-ecological sampling approach to judgment biases. Psychol. Rev. 107, 659–676 (2000).
- 62.
Fiedler, K. & Juslin, P. Information Sampling and Adaptive Cognition (Cambridge Univ. Press, 2006).
- 63.
Gigerenzer, G., Hertwig, R. & Pachur, T. Heuristics: The Foundations of Adaptive Behavior (Oxford Univ. Press, 2011).
- 64.
Pachur, T., Hertwig, R. & Rieskamp, J. Intuitive judgments of social statistics: how exhaustive does sampling need to be? J. Exp. Soc. Psychol. 49, 1059–1077 (2013).
- 65.
Simon, H. A. Models of Man; Social and Rational (Wiley, 1957).
- 66.
Hertwig, R. & Hoffrage, U. Simple Heuristics in a Social World (Oxford Univ. Press, 2013).
- 67.
Schulze, C., Hertwig, R. & Pachur, T. Who you know is what you know: modeling boundedly rational social sampling. J. Exp. Psychol. Gen. 150, 221–241 (2021).
- 68.
McPherson, M., Smith-Lovin, L. & Cook, J. M. Birds of a feather: homophily in social networks. Annu. Rev. Sociol. 27, 415–444 (2001).
- 69.
Galesic, M., Olsson, H. & Rieskamp, J. A sampling model of social judgment. Psychol. Rev. 125, 363–390 (2018). Develops a computational model of social judgment based on people’s perceptions of their immediate social environment.
- 70.
Frable, D. E. S. Being and feeling unique: statistical deviance and psychological marginality. J. Pers. 61, 85–110 (1993).
- 71.
Kruger, J. Lake Wobegon be gone! The “below-average effect” and the egocentric nature of comparative ability judgments. J. Pers. Soc. Psychol. 77, 221–232 (1999).
- 72.
Kruger, J. & Dunning, D. Unskilled and unaware of it: how difficulties in recognizing one’s own incompetence lead to inflated self-assessments. J. Pers. Soc. Psychol. 77, 1121–1134 (1999).
- 73.
Sudman, S. & Kalton, G. New developments in the sampling of special populations. Annu. Rev. Sociol. 12, 401–429 (1986).
- 74.
Feld, S. L. Why your friends have more friends than you do. Am. J. Sociol. 96, 1464–1477 (1991). Introduces the friendship paradox, the phenomenon in which the mean number of friends of friends is always greater than the mean number of friends of individuals.
- 75.
Cohen, R., Havlin, S. & Ben-Avraham, D. Efficient immunization strategies for computer networks and populations. Phys. Rev. Lett. 91, 247901 (2003).
- 76.
Kumar, V., Krackhardt, D. & Feld, S. Interventions with inversity in unknown networks can help regulate contagion. Preprint at https://arxiv.org/abs/2105.08758 (2021). Based on the logic underlying the friendship paradox, this paper develops strategies to use reports from random individuals to identify better connected individuals in networks where the overall structures are unknown or evolving.
- 77.
Kim, D. A. et al. Social network targeting to maximise population behaviour change: a cluster randomised controlled trial. Lancet 386, 145–153 (2015).
- 78.
Aggarwal, C. C. & Abdelzaher, T. in Managing and Mining Sensor Data 237–297 (Springer, 2013).
- 79.
Szell, M., Lambiotte, R. & Thurner, S. Multirelational organization of large-scale social networks in an online world. Proc. Natl Acad. Sci. USA 107, 13636–13641 (2010).
- 80.
Centola, D. & Macy, M. Complex contagions and the weakness of long ties. Am. J. Sociol. 113, 702–734 (2007).
- 81.
Guilbeault, D., Baronchelli, A. & Centola, D. Experimental evidence for scale-induced category convergence across populations. Nat. Commun. 12, 327 (2021).
- 82.
Bruch, E. & Mare, R. D. Neighborhood choice and neighborhood change. Am. J. Sociol. 112, 667–709 (2006).
- 83.
Bruch, E., Feinberg, F. & Lee, K. Y. Extracting multistage screening rules from online dating activity data. Proc. Natl Acad. Sci. USA 113, 10530–10535 (2016).
- 84.
Yang, V. C., Abrams, D. M., Kernell, G. & Motter, A. E. Why are U.S. parties so polarized? A “satisficing” dynamical model. SIAM Rev. 62, 646–657 (2020).
- 85.
Dalege, J. et al. Toward a formalized account of attitudes: The Causal Attitude Network (CAN) model. Psychol. Rev. 123, 2–22 (2016). Introduces a measurement model of attitudes relying on network principles.
- 86.
Jackson, M. O. Social and Economic Networks (Princeton Univ. Press, 2010).
- 87.
Jędrzejewski, A. & Sznajd-Weron, K. Statistical physics of opinion formation: is it a SPOOF? C. R. Phys. 20, 244–261 (2019).
- 88.
Redner, S. Reality-inspired voter models: a mini-review. C. R. Phys. 20, 275–292 (2019).
- 89.
Simon, H. A. Invariants of human behavior. Annu. Rev. Psychol. 41, 1–20 (1990).
- 90.
Durkheim, E. The Division of Labour in Society (trans. Simpson, G.) (Free Press, 1893).
- 91.
Thomas, W. I. & Swaine Thomas, D. The Child in America: Behaviour Problems and Programs (Knopf, 1928).
- 92.
DiMaggio, P. Culture and cognition. Annu. Rev. Sociol. 23, 263–287 (1997).
- 93.
Johnson, C., Dowd, T. J. & Ridgeway, C. L. Legitimacy as a social process. Annu. Rev. Sociol. 32, 53–78 (2006).
- 94.
Newman, M. E. J. Network structure from rich but noisy data. Nat. Phys. 14, 542–545 (2018).
- 95.
Smith, E. R. & Zárate, M. A. Exemplar-based model of social judgment. Psychol. Rev. 99, 3–21 (1992).
- 96.
Denrell, J. Why most people disapprove of me: experience sampling in impression formation. Psychol. Rev. 112, 951–978 (2005).
- 97.
Gonzalez, C., Ben-Asher, N., Martin, J. M. & Dutt, V. A cognitive model of dynamic cooperation with varied interdependency information. Cogn. Sci. 39, 457–495 (2015).
- 98.
Cialdini, R. B. & Goldstein, N. J. Social influence: compliance and conformity. Annu. Rev. Psychol. 55, 591–621 (2004).
- 99.
Efferson, C., Lalive, R., Richerson, P. J., McElreath, R. & Lubell, M. Conformists and mavericks: the empirics of frequency-dependent cultural transmission. Evol. Hum. Behav. 29, 56–64 (2008).
- 100.
McElreath, R., Wallin, A. & Fasolo, B. in Simple Heuristics in a Social World (eds Hertwig, R. & Hoffrage, U.) 381–408 (Oxford Univ. Press, 2013).
- 101.
Tump, A. N., Pleskac, T. J. & Kurvers, R. H. J. M. Wise or mad crowds? The cognitive mechanisms underlying information cascades. Sci. Adv. 6, eabb0266 (2020).
- 102.
Moussaïd, M., Kämmer, J. E., Analytis, P. P. & Neth, H. Social influence and the collective dynamics of opinion formation. PLoS One 8, e78433 (2013).
- 103.
Analytis, P. P., Barkoczi, D. & Herzog, S. M. Social learning strategies for matters of taste. Nat. Hum. Behav. 2, 415–424 (2018).
- 104.
Molleman, L. et al. Strategies for integrating disparate social information. Proc. R. Soc. Lond. B 287, 20202413 (2020).
- 105.
Boyd, R. & Richerson, P. J. Culture and the Evolutionary Process (Univ. Chicago Press, 1985).
- 106.
Degroot, M. H. Reaching a consensus. J. Am. Stat. Assoc. 69, 118–121 (1974).
- 107.
Condorcet, M. Essai sur l’Application de l’Analyse à la Probabilité des Décisions Rendues à la Pluralité des Voix [Essay on the Application of Analysis to the Probability of Majority Decisions] (Imprimerie Royale, 1785).
- 108.
Krapivsky, P. L. & Redner, S. Dynamics of majority rule in two-state interacting spin systems. Phys. Rev. Lett. 90, 238701 (2003).
- 109.
Hoppitt, W. & Laland, K. N. Social Learning: An Introduction to Mechanisms, Methods, and Models (Princeton Univ. Press, 2013).
- 110.
Galesic, M. & Stein, D. L. Statistical physics models of belief dynamics: theory and empirical tests. Phys. A Stat. Mech. Appl. 519, 275–294 (2019).
- 111.
Marr, D. Vision (Freeman, 1982).
- 112.
Anderson, J. R. The Adaptive Character of Thought (Lawrence Erlbaum, 1990).
- 113.
Chater, N., Tenenbaum, J. B. & Yuille, A. Probabilistic models of cognition: conceptual foundations. Trends Cogn. Sci. 10, 287–291 (2006).
- 114.
Baker, C., Saxe, R., Tenenbaum, J. & Baker, C. L. Bayesian theory of mind: modeling joint belief-desire attribution. In Proc. Annual Meeting of the Cognitive Science Society 2469–2474 (2011).
- 115.
Krafft, P. M., Shmueli, E., Griffiths, T. L., Tenenbaum, J. B. & Pentland, A. S. Bayesian collective learning emerges from heuristic social learning. Cognition 212, 104469 (2021).
- 116.
Baltag, A., Christoff, Z., Rendsvig, R. K. & Smets, S. Dynamic epistemic logics of diffusion and prediction in social networks. Stud. Log. 107, 489–531 (2019).
- 117.
Schweighofer, S., Schweitzer, F. & Garcia, D. A weighted balance model of opinion hyperpolarization. JASSS 23, 5 (2020). Proposes a model of belief change based on balanced networks accounting for the importance of related issues and other individuals.
- 118.
Schweitzer, F., Krivachy, T. & Garcia, D. An agent-based model of opinion polarization driven by emotions. Complexity 2020, 1–11 (2020).
- 119.
Castellano, C., Fortunato, S. & Loreto, V. Statistical physics of social dynamics. Rev. Mod. Phys. 81, 591–646 (2009). Reviews many models of social dynamics inspired by analogies from statistical physics.
- 120.
Perc, M. et al. Statistical physics of human cooperation. Phys. Rep. 687, 1–51 (2017).
- 121.
Dalege, J., Borsboom, D., van Harreveld, F. & van der Maas, H. L. J. The attitudinal entropy (AE) framework as a general theory of individual attitudes. Psychol. Inq. 29, 175–193 (2018). Develops a general theory on individual attitudes using a statistical physics framework.
- 122.
Minh Pham, T., Kondor, I., Hanel, R. & Thurner, S. The effect of social balance on social fragmentation. J. R. Soc. Interface 17, 20200752 (2020).
- 123.
Rodriguez, N., Bollen, J. & Ahn, Y.-Y. Collective dynamics of belief evolution under cognitive coherence and social conformity. PLoS One 11, e0165910 (2016). Proposes a belief change model that combines social and cognitive factors as interacting networks driven towards stable triads.
- 124.
Salganik, M. J. Bit by Bit: Social Research in the Digital Age (Princeton Univ. Press, 2018).
- 125.
Pikler, A. G. Utility theories in field physics and mathematical economics (I). Br. J. Philos. Sci. 5, 47–58 (1954).
- 126.
Festinger, L. A Theory of Cognitive Dissonance (Stanford Univ. Press, 1957).
- 127.
Lewenstein, M., Nowak, A. & Latané, B. Statistical mechanics of social impact. Phys. Rev. A 45, 763–776 (1992).
- 128.
Morone, F. & Makse, H. A. Influence maximization in complex networks through optimal percolation. Nature 524, 65–68 (2015).
- 129.
Haven, E. & Khrennikov, A. Quantum Social Science (Cambridge Univ. Press, 2013).
- 130.
Bettencourt, L. M. A., Cintrón-Arias, A., Kaiser, D. I. & Castillo-Chávez, C. The power of a good idea: quantitative modeling of the spread of ideas from epidemiological models. Phys. A Stat. Mech. Appl. 364, 513–536 (2006).
- 131.
Daley, D. J. & Kendall, D. G. Epidemics and rumours. Nature 204, 1118 (1964).
- 132.
Henrich, J. & McElreath, R. The evolution of cultural evolution. Evol. Anthropol. 12, 123–135 (2003).
- 133.
Holyoak, K. J. & Thagard, P. Mental Leaps: Analogy in Creative Thought (MIT Press, 1995).
- 134.
Gigerenzer, G. From tools to theories: a heuristic of discovery in cognitive psychology. Psychol. Rev. 98, 254–267 (1991).
- 135.
Dasgupta, A., Kumar, R. & Sivakumar, D. Social sampling. In Proc. 18th ACM SIGKDD International Conference on Knowledge Discovery and Data Mining 235 (ACM Press, 2012).
- 136.
Nettasinghe, B. & Krishnamurthy, V. ‘What do your friends think?’: efficient polling methods for networks using friendship paradox. IEEE Trans. Knowl. Data Eng. 33, 1291–1305 (2019).
- 137.
Kim, D., Gile, K. J., Guarino, H. & Mateu‐Gelabert, P. Inferring bivariate association from respondent‐driven sampling data. J. R. Stat. Soc. Ser. C 70, 415–433 (2021).
- 138.
Gile, K. J. & Handcock, M. S. Respondent-driven sampling: an assessment of current methodology. Sociol. Methodol. 40, 285–327 (2010).
- 139.
Kim, B. J. & Handcock, M. S. Population size estimation using multiple respondent-driven sampling surveys. J. Surv. Stat. Methodol. 9, 94–120 (2021).
- 140.
Berchenko, Y., Rosenblatt, J. D. & Frost, S. D. W. Modeling and analyzing respondent-driven sampling as a counting process. Biometrics 73, 1189–1198 (2017).
- 141.
Crawford, F. W., Wu, J. & Heimer, R. Hidden population size estimation from respondent-driven sampling: a network approach. J. Am. Stat. Assoc. 113, 755–766 (2018).
- 142.
Klingwort, J., Buelens, B. & Schnell, R. Capture–recapture techniques for transport survey estimate adjustment using permanently installed highway-sensors. Soc. Sci. Comput. Rev. https://doi.org/10.1177/0894439319874684 (2019).
- 143.
Tourangeau, R., Rips, L. T. & Rasinski, K. The Psychology of Survey Response (Cambridge Univ. Press, 2000).
- 144.
Batchelder, W. H. & Romney, A. K. Test theory without an answer key. Psychometrika 53, 71–92 (1988).
- 145.
Romney, A. K., Weller, S. C. & Batchelder, W. H. Culture as consensus: a theory of culture and informant accuracy. Am. Anthropol. 88, 313–338 (1986).
- 146.
Prelec, D. A Bayesian truth serum for subjective data. Science 306, 462–466 (2004). Develops a Bayesian algorithm that incentivizes honest answers in a survey even if honesty is not independently verifiable.
- 147.
Miller, N., Resnick, P. & Zeckhauser, R. Eliciting informative feedback: the peer-prediction method. Manage. Sci. 51, 1359–1373 (2005).
- 148.
Baillon, A. Bayesian markets to elicit private information. Proc. Natl Acad. Sci. USA 114, 7958–7962 (2017).
- 149.
Cvitanić, J., Prelec, D., Riley, B. & Tereick, B. Honesty via choice-matching. Am. Econ. Rev. Insights 1, 179–192 (2019).
- 150.
John, L. K., Loewenstein, G. & Prelec, D. Measuring the prevalence of questionable research practices with incentives for truth telling. Psychol. Sci. 23, 524–532 (2012).
- 151.
Prelec, D., Seung, H. S. & McCoy, J. A solution to the single-question crowd wisdom problem. Nature 541, 532–535 (2017). Addressing Galton’s original crowd wisdom problem (Nature 1907), this study provides a Bayesian criterion that identifies correct answers to a multiple choice questions even if the majority of polled individuals are wrong.
- 152.
Foster, I., Ghani, R., Jarmin, R. S., Kreuter, F. & Lane, J. Big Data and Social Science: A Practical Guide to Methods and Tools (CRC Press, 2017). Provides practical guidance on combining methods and tools from computer science, statistics, and social science.
- 153.
Lane, J. Democratizing Our Data: A Manifesto (MIT Press, 2020).
- 154.
Haas, G. C., Trappmann, M., Keusch, F., Bähr, S. & Kreuter, F. Using geofences to collect survey data: lessons learned from the IAB-SMART study. Surv. Methods Insights Field https://doi.org/10.13094/SMIF-2020-00023 (2020).
- 155.
Christen, P., Ranbaduge, T. & Schnell, R. Linking Sensitive Data: Methods and Techniques for Practical Privacy-Preserving Information Sharing (Springer, 2020).
- 156.
Couper, M. P. Is the sky falling? New technology, changing media, and the future of surveys. Surv. Res. Methods 7, 145–156 (2013).
- 157.
Hill, C. et al. Big Data Meets Survey Science: A Collection of Innovative Methods (Wiley, 2020).
- 158.
Schnell, R. Survey-Interviews: Methoden standardisierter Befragungen (Springer, 2019).
- 159.
Olsson, H., Barman-Adhikari, A., Galesic, M., Hsu, H.-T. & Rice, E. Cognitive strategies for peer judgments. Preprint at https://doi.org/10.31219/osf.io/s3hxj (2021).
- 160.
van der Maas, H. L. J., Dalege, J. & Waldorp, L. The polarization within and across individuals: the hierarchical Ising opinion model. J. Complex Netw. 8, cnaa010 (2020).
- 161.
Fails, J. A. & Olsen, D. R. Interactive machine learning. in Proc. 8th International Conference on Intelligent User Interfaces 39 (ACM Press, 2003).
- 162.
Jiang, L., Liu, S. & Chen, C. Recent research advances on interactive machine learning. J. Vis. 22, 401–417 (2019).
- 163.
Ware, M., Frank, E., Holmes, G., Hall, M. & Witten, I. H. Interactive machine learning: letting users build classifiers. Int. J. Hum. Comput. Stud. 55, 281–292 (2001).
- 164.
Fortuna, P. & Nunes, S. A survey on automatic detection of hate speech in text. ACM Comput. Surv. 51, 1–30 (2018).
- 165.
Garland, J., Ghazi-Zahedi, K., Young, J.-G., Hébert-Dufresne, L. & Galesic, M. Impact and dynamics of hate and counter speech online. Preprint at https://arxiv.org/abs/2009.08392 (2020).
- 166.
Leader Maynard, J. & Benesch, S. Dangerous speech and dangerous ideology: an integrated model for monitoring and prevention. Genocide Stud. Prev. 9, 70–95 (2016).
- 167.
Abeliuk, A., Benjamin, D. M., Morstatter, F. & Galstyan, A. Quantifying machine influence over human forecasters. Sci. Rep. 10, 15940 (2020).
- 168.
Huber, D. J. et al. MATRICS: A system for human-machine hybrid forecasting of geopolitical events. In 2019 IEEE Intl Conf. Big Data 2028–2032 (IEEE, 2019).
- 169.
Morstatter, F. et al. SAGE: a hybrid geopolitical event forecasting system. In Intl Joint Conf. Artificial Intelligence 6557–6559 (2019).
- 170.
Evans, J. Social computing unhinged. J. Soc. Comput. 1, 1–13 (2020).
- 171.
Wagner, C. et al. Measuring algorithmically infused societies. Nature https://doi.org/10.1038/s41586-021-03666-1 (2021).
- 172.
Hidalgo, C. A., Orghiain, D., Canals, J. A., De Almeida, F. & Martín, N. How Humans Judge Machines (MIT Press, 2021).
- 173.
Rahwan, I. et al. Machine behaviour. Nature 568, 477–486 (2019).
- 174.
Lazer, D. et al. Meaningful measures of human society in the twenty-first century. Nature https://doi.org/10.1038/s41586-021-03660-7 (2021).
- 175.
Hofman, J. M. et al. Integrating explanation and prediction in computational social science. Nature https://doi.org/10.1038/s41586-021-03659-0 (2021).
- 176.
Trouille, L., Lintott, C. J. & Fortson, L. F. Citizen science frontiers: Efficiency, engagement, and serendipitous discovery with human-machine systems. Proc. Natl Acad. Sci. USA 116, 1902–1909 (2019).
- 177.
Outbreaks near me; https://outbreaksnearme.org/us/en-US/
- 178.
Schurz, M. et al. Toward a hierarchical model of social cognition: a neuroimaging meta-analysis and integrative review of empathy and theory of mind. Psychol. Bull. 147, 293–327 (2021).
- 179.
Adolphs, R. Cognitive neuroscience of human social behaviour. Nat. Rev. Neurosci. 4, 165–178 (2003).
- 180.
Feng, C. et al. Common brain networks underlying human social interactions: evidence from large-scale neuroimaging meta-analysis. Neurosci. Biobehav. Rev. 126, 289–303 (2021).
- 181.
Estes, W. K. Classification and Cognition (Oxford Univ. Press, 1994).
- 182.
Rieskamp, J. & Hoffrage, U. Inferences under time pressure: how opportunity costs affect strategy selection. Acta Psychol. 127, 258–276 (2008).
- 183.
Ambady, N. & Rosenthal, R. Thin slices of expressive behavior as predictors of interpersonal consequences: a meta-analysis. Psychol. Bull. 111, 256–274 (1992).
- 184.
Jern, A. & Kemp, C. A decision network account of reasoning about other people’s choices. Cognition 142, 12–38 (2015).
- 185.
Zacks, R. T. & Hasher, L. in Etc. Frequency Processing and Cognition Vol. 6, 21–36 (Oxford Univ. Press, 2002).
- 186.
Conrad, F. G., Brown, N. R. & Cashman, E. R. Strategies for estimating behavioural frequency in survey interviews. Memory 6, 339–366 (1998).
- 187.
Lynn, C. W. & Bassett, D. S. How humans learn and represent networks. Proc. Natl Acad. Sci. USA 117, 29407–29415 (2020).
- 188.
Gagné, F. M. & Lydon, J. E. Bias and accuracy in close relationships: an integrative review. Pers. Soc. Psychol. Rev. 8, 322–338 (2004).
- 189.
Goel, S., Mason, W. & Watts, D. J. Real and perceived attitude agreement in social networks. J. Pers. Soc. Psychol. 99, 611–621 (2010).
- 190.
Dawes, R. M. Statistical criteria for establishing a truly false consensus effect. J. Exp. Soc. Psychol. 25, 1–17 (1989).
- 191.
Nisbett, R. E. & Kunda, Z. Perception of social distributions. J. Pers. Soc. Psychol. 48, 297–311 (1985).
- 192.
Galesic, M., Olsson, H. & Rieskamp, J. Social sampling explains apparent biases in judgments of social environments. Psychol. Sci. 23, 1515–1523 (2012).
- 193.
Killworth, P. D. & Bernard, H. Informant accuracy in social network data. Hum. Organ. 35, 269–286 (1976).
- 194.
Bernard, H. R. & Killworth, P. D. Informant accuracy in social network data II. Hum. Commun. Res. 4, 3–18 (1977).
- 195.
Freeman, L. C., Romney, A. K. & Freeman, S. C. Cognitive structure and informant accuracy. Am. Anthropol. 89, 310–325 (1987).
- 196.
Chang, L. & Krosnick, J. A. Measuring the frequency of regular behaviors: comparing the “typical week” to the “past week”. Sociol. Methodol. 33, 55–80 (2003).
- 197.
Feld, S. L. & Carter, W. C. Detecting measurement bias in respondent reports of personal networks. Soc. Networks 24, 365–383 (2002).
- 198.
Banerjee, A. V., Chandrasekhar, A. G., Duflo, E. & Jackson, M. O. Using gossips to spread information: theory and evidence from two randomized controlled trials. MIT Department of Economics Working Paper No. 14–15 http://www.ssrn.com/abstract=2425379 (2014).
Acknowledgements
We thank F. Gerdon, J. Foster, R. Kurvers, M. Schierholz, P. Schenk, T. Wallsten, and C. Wagner for comments on an earlier version of the manuscript, as well as our many collaborators for their contributions to this work. M.G., H.O., T.v.d.D., J.D., W.B.d.B., and D.P. were supported in part by grants from the National Science Foundation (M.G.: DRMS-1757211; H.O., M.G., and J.D.: BCS-1918490; M.G., H.O., and T.v.d.D.: DRMS-1949432; H.O., M.G., W.B.d.B., and D.P.: MMS-2019982), M.G., T.v.d.D., and D.L.S. were supported in part by a grant from the National Institute of Food and Agriculture (NIFA 2018-67023-27677), and J.D. was supported in part by an EU Horizon 2020 Marie Curie Global Fellowship (no. 889682).
Author information
Affiliations
Contributions
All authors contributed equally to the writing of this Perspective.
Corresponding author
Ethics declarations
Competing interests
The authors declare no competing interests.
Additional information
Peer review information Nature thanks Jacob Foster, Ralf Kurvers and the other, anonymous, reviewer(s) for their contribution to the peer review of this work.
Publisher’s note Springer Nature remains neutral with regard to jurisdictional claims in published maps and institutional affiliations.
Rights and permissions
About this article
Cite this article
Galesic, M., Bruine de Bruin, W., Dalege, J. et al. Human social sensing is an untapped resource for computational social science. Nature (2021). https://ift.tt/3hrkOxY
-
Received:
-
Accepted:
-
Published:
Comments
By submitting a comment you agree to abide by our Terms and Community Guidelines. If you find something abusive or that does not comply with our terms or guidelines please flag it as inappropriate.
"social" - Google News
June 30, 2021 at 10:01PM
https://ift.tt/3h2Hl5n
Human social sensing is an untapped resource for computational social science - Nature.com
"social" - Google News
https://ift.tt/38fmaXp
https://ift.tt/2WhuDnP
Bagikan Berita Ini
0 Response to "Human social sensing is an untapped resource for computational social science - Nature.com"
Post a Comment